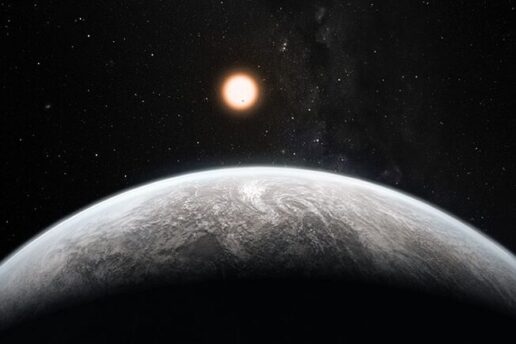
Research updates from TESS: Hunting for worlds beyond our solar system
MIT scientists, including physics grad student Rahul Jayaraman, present exoplanet data at the 237th American Astronomical Society meeting.
At the annual meeting of the American Astronomical Society (AAS) held last month, MIT researchers presented new data from programs such as the Transiting Exoplanet Survey Satellite (TESS). In a virtual-only format, scientists from the MIT Department of Earth, Atmospheric and Planetary Sciences, the Department of Physics, and the MIT Kavli Institute for Astrophysics and Space Research shared their findings, including using atmospheric characterization and machine learning tools and techniques to learn more about extrasolar planets.
Beyond the sun
For researchers interested in planets beyond our solar system, the universe’s infinite array of stars and planets offer too many targets for study. How can scientists possibly narrow down the options and determine which planets to focus on?
Enter TESS: a NASA mission led by MIT researchers. On April 18, 2018, the space telescope began its two-year primary mission to image large swaths of the sky and allow researchers to look for planets orbiting other stars. TESS completed this mission last summer, having imaged three-quarters of the sky and enabled the identification of 66 new exoplanets and 2,100 planetary candidates. For the next two years, TESS will collect information across the sky with finer data resolution, beginning with the southern hemisphere.
At the AAS meeting, MIT scientists shared their TESS findings with conference attendees, while looking forward to what will come as the telescope begins its extended mission.
Atmospheric characterization
To identify potential exoplanets with TESS, researchers look for changes in the amount of light coming from a star. A small dip in a star’s light might mean that a planet has passed in front of it, blocking some of its light from reaching Earth. By measuring these so-called transits, scientists can approximate the size of a planet, how long it takes to orbit its star, and whether it has other planetary neighbors.
Ian Wong, a 51 Pegasi b Postdoctoral Fellow in MIT’s Department of Earth, Atmospheric and Planetary Sciences, presented his observations of a wide range of exoplanets observed by TESS’s primary mission.
Wong used TESS data to observe 18 known exoplanets passing in front of their host stars, but he was also interested in what happened when they traveled behind their stars. Termed a “secondary eclipse,” the small dip in light that occurs when a planet is blocked by its star reveals how much and what kind of light a planet reflects or emits. This enables scientists to learn about the albedo (how much light it reflects) and temperature of the side of an exoplanet directly facing its star.
Through his two-year project, Wong found that as a planet’s dayside temperature increases from 2,200 degrees Fahrenheit to 5,000 F, so does its albedo. This is a surprising trend, since clouds — which contribute significantly to a planet’s albedo — are unlikely to form at the upper end of this high temperature range. However, said Wong, it’s possible that clouds from the nightside of the planet could move to the dayside or that the light is reflected by another substance, like bright-white titanium dioxide. This correlation is still tentative, and Wong will investigate it further with upcoming data from TESS’ extended mission.
Applications for machine learning
Maximilian N. Günther is a Juan Carlos Torres Fellow and postdoc at MIT. On the first day of the conference, Günther presented a talk in which he showed how he has used machine learning to measure stellar flares (explosive events from stars), which could be indications of habitable planets.
His talk addressed the impact of star flares and coronal mass ejections, or large releases of plasma into solar wind, on the search for habitable planets. It’s essential to map stellar flares, because they may do one of two things: either strip an atmosphere of the elements needed for life, or trigger energy for the chemistry that can eventually lead to life. Flaring can indicate planet type, age, and other important factors.
As a young planet develops, Günther said, “You want extreme flaring that can form these precursors of RNA and prebiotic chemistry. But once this is triggered, you basically want the flaring to stop, because you want life to develop and for the ozone not to be depleted anymore. So it’s this fine, sweet spot.”
Machine learning occurs when algorithms can quickly sift through and make sense of massive amounts of data. Advanced machine learning has allowed for an efficient, detailed report of the stellar flares that TESS captured in its first two years. Without machine learning, the process would’ve taken more than a lifetime to complete.
Günther also emphasized that while using machine learning to examine TESS data can help identify potentially inhabitable planets, “if we don’t see the right amount of flaring, that doesn’t necessarily rule out that there might not be other things like volcanism, lightning strikes, or hydrothermal vents triggering life on those planets. So this is basically just one pathway of life that I’m looking at.”
Rahul Jayaraman is a second-year PhD student at MIT specializing in large-scale searches of the TESS data for intriguing phenomena. Jayaraman presented his work, “Leveraging Machine Learning to Detect Transients in TESS FFIs.” FFIs are full-frame images, detailed photographs produced by TESS spanning its entire field of view.
While TESS primarily exists to detect exoplanets, TESS’ ability to image the entire sky may also allow researchers to identify short-lived, or transient, events. These events may include supernovae and afterglows from gamma ray bursts — highly energetic, strong bursts of energy believed to occur during black hole formation.
“TESS looks at a large region of the sky for a continuous period of time,” Jayaraman explained during the talk. “We expect that analyzing the time series data from all those FFIs might yield pixels that show significant variability, which could potentially correspond to astrophysical transient events.”
Jayaraman’s group is working to design an algorithm that could identify pixels from TESS’s FFIs that may indicate rare and exciting transient events –– events that might not otherwise be observed.
Ultimately, the researchers hope that the algorithm will function without human supervision. Ideally, the program will narrow down thousands of potentially rare events to just a few dozen or so for a scientist to study.
“I think [for now] there should still be a human just to make sure that there aren’t any false positives that are sent out,” Jayaraman said during his talk. “But I think the end goal really is to make sure that we can get something that’s sort of fully automated. Once we have increased confidence in our code, we can work on that.”
TESS is a NASA Astrophysics Explorer mission led and operated by MIT in Cambridge, Massachusetts, and managed by NASA’s Goddard Space Flight Center. Additional partners include Northrop Grumman, based in Falls Church, Virginia; NASA’s Ames Research Center in California’s Silicon Valley; the Center for Astrophysics – Harvard and Smithsonian in Cambridge; MIT Lincoln Laboratory; and the Space Telescope Science Institute in Baltimore. More than a dozen universities, research institutes, and observatories worldwide are participants in the mission.
“Leveraging Machine Learning to Detect Transients in TESS FFIs.”