Quantum Information Science
There is a worldwide research effort exploring the potentials of quantum mechanics for applications. The field began with Feynman’s proposal in 1981 at MIT Endicott House to build a computer that takes advantage of quantum mechanics and has grown enormously since Peter Shor’s 1994 quantum factoring algorithm. The idea of utilizing quantum mechanics to process information has since grown from computation and communication to encompass diverse topics such as sensing and simulations in biology and chemistry. Leaving aside the extensive experimental efforts to build controllable large-scale quantum devices, theory research in quantum information science (QIS) investigates several themes:
- Quantum algorithms and complexity
- If a perfectly functioning quantum computer were built, which problems could it solve faster than conventional computers, and which problems do not admit any speedup?
- What is the fundamental limitation in the computational power of our universe governed by quantum mechanics?
- Quantum information theory
- How can we store, transfer, or manipulate quantum information in the presence of noise?
- How can we efficiently communicate and compute in the presence of errors?
- What are the basic properties of quantum entanglement and information?
- Measurement and control
- How can we efficiently manipulate and characterize quantum devices?
- Apart from computations and communications, what other applications can benefit from quantum hardwares?
- What are the ways to improve applications such as ultra-sensitive sensors or precise clocks?
- Applications and connections:
- How can ideas from QIS contribute to other research areas as diverse as convex optimizations, black holes, and exotic quantum phases of matter?
- Is there a unified framework to efficiently describe quantum entanglement and information in complex systems?
QIS theory research at MIT spans all of these areas. The CTP faculty involved are: Soonwon Choi and Aram Harrow, and the larger group at MIT includes Isaac Chuang (EECS/physics), Seth Lloyd (Mech. Eng.), Anand Natarajan (EECS) and Peter Shor (Math). Other faculty in the area include Eddie Farhi (emeritus), Jeffrey Goldstone (emeritus) and Jeff Shapiro (EECS, emeritus). Together this forms a large and vibrant group working in all areas of QIS.
Some of the notable contributions involving the CTP include the quantum adiabatic algorithm and quantum walk algorithms (Farhi, Goldstone), the first example of a problem for which quantum computers exhibit no speedup (Farhi, Goldstone), proposals for unforgeable quantum money (Farhi, Shor), a quantum algorithm for linear systems of equations (Harrow, Lloyd), efficient protocols for simulating quantum channels (Harrow, Shor), both algorithms and hardness results for testing entanglement (Harrow), proposals for quantum approximate optimization algorithms (Farhi, Goldstone), proposals and experimental observations of exotic quantum dynamics such as slow thermalization or a discrete time crystalline phase in quantum simulators (Choi), quantum sensing protocols using strongly interacting spin ensembles (Choi), and quantum convolutional neural networks (Choi). Ongoing research at MIT in QIS includes work on new quantum algorithms, efficient simulations of quantum systems, methods to characterize and control existing or near-term quantum hardwares, connections to many-body physics, applications in high-energy physics, and many other topics.
The larger QIS group at MIT shares a seminar series, a weekly group meeting, regular events for grad students.
Interdepartmental course offerings include an introductory and an advanced class in core QI/QC, as well as occasional advanced special topics classes. Quantum information has also entered the undergraduate physics curriculum with a junior lab experiment on NMR quantum computing and some lectures in the 8.04/8.05/8.06 sequence on quantum computing.
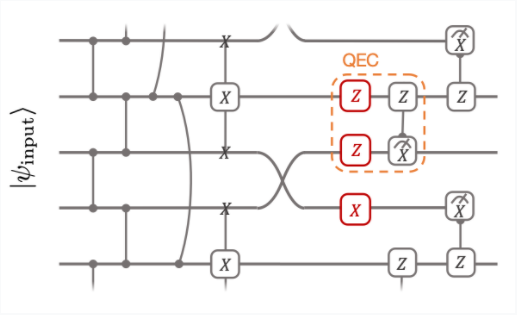
Credit: Adapted from I.Cong, S.Choi, M.D.Lukin,
Nature Physics volume 15, 1273–1278 (2019)